This article is a part of a Statistics Canada and CIGI collaboration to discuss data needs for a changing world.
“Official statistics provide an indispensable element in the information system of a democratic society.” This is first among the United Nations’ Fundamental Principles of Official Statistics. Accordingly, national statistical offices (NSOs) gather, guard and grow data to provide information and insights to society using an array of methods and approaches. These methods have traditionally revolved around survey sampling and sometimes been supplemented by administrative data files to improve some elements of quality.
As the world changes, the methods and techniques used to measure economic, societal and environmental phenomena have necessarily evolved. NSOs have historically managed the evolution of statistical methods quite well. However, in recent years, the data landscape has been shifting at an incredible pace and scale. This rapid evolution, combined with digitization and technological advances, has really contributed to the democratization of data. There are many new players in the data game, and data collection and dissemination are no longer the purview of NSOs only. Individuals, businesses and governments are consuming and producing information at an unprecedented rate. The demands for information are no longer satisfied by annual, quarterly or even monthly data reports, the typical suite of traditional official statistics provided by NSOs. Society is now demanding data in real time. Yet, data must be available at a much finer level of detail and broken down into smaller socio-demographic subpopulations (disaggregated data), including information about its most vulnerable populations, to address the information needs of society. Further, the time required for planning, developing and implementing traditional methods often does not align with the fast-paced agendas of those who need the information.
As the world of data becomes more complex, the need to better manage it through good data governance and well-articulated strategies becomes more urgent. As Anil Arora and Rohinton P. Medhora wrote recently, the world needs solid data stewardship. In this context, NSOs must strengthen their current methods but also explore and embrace new methods and approaches to be ready to measure society as it changes. Further, it is important that they be organized so that their services can meet user needs. This article explores a wide array of modern collection methods that provide NSOs with a continuum of options to employ in their work of quality, rigorous and transparent data collection.
Meeting Needs with Modern Methods
Data needs have evolved over time, and the data revolution and, more importantly, the coronavirus disease 2019 (COVID-19) pandemic, have catalyzed the development, testing and implementation of new methods. These, combined with established approaches, now help NSOs to better fulfill society’s data needs by enabling the production of statistical measures that go beyond the traditional statistics for which they are known. As Principle 1 of the United Nations’ Fundamental Principles goes on to say, “official statistics that meet the test of practical utility are to be compiled” — highlighting that it is crucial to renew the programs in order to maintain statistics’ usefulness. Indeed, one of Canada’s former chief statisticians, Ivan P. Fellegi, takes it further, arguing that preserving the spirit of research and innovation is a survival issue: “Simply carrying on with the same programme…is a recipe for eventual irrelevance.”
Motivated by this new data context, Statistics Canada embarked on a modernization agenda in 2017. The agenda takes a user-centric approach, that is, one focused on answering the needs of data users. To achieve this objective, one of the initiative’s foundational pillars is to aim to be at the leading edge of statistical techniques and methods. Building on a deep culture of science, Statistics Canada decided to explicitly adopt the scientific approach, which starts with an expressed need; from there, hypotheses are formulated, based on available knowledge and a review of the literature, and tested. Conclusions are derived from the results and the hypotheses can thus be updated. This cycle continues, with researchers all the while keeping the process and results transparent to their peers. As its latest departmental plan describes, Statistics Canada is employing this approach in its proactive study and testing of new methods of data collection.
In the early stages of its modernization, Statistics Canada identified four “pathfinder projects” that it would develop incrementally, using new collection methods. These projects would, respectively, measure the effects of legalization of cannabis; establish new partnerships with data providers to build a comprehensive source of housing information; increase the availability of data on clean technology in Canada; and integrate new sources of data with traditional ones to produce enhanced tourism indicators.
For example, when Canada was preparing to legalize cannabis and needed data on cannabis consumption, Statistics Canada had to quickly implement a program to measure the social and economic impacts of cannabis legalization. This pathfinder project used crowdsourcing to collect data from the general public anonymously. In another of the pathfinder projects, the Canadian Housing Statistics Program used a new administrative-first approach, whereby instead of carrying out surveys first and possibly adding administrative data to complement them, it first started with administrative data and then considered complementing that data with surveys. With this new approach, it began producing estimates in some areas of Canada where the methods and availability of data sources enabled it, increasing the coverage through iterations of the program.
One family of approaches and methods that has particularly contributed to this evolution is data science, which brings new methods, techniques and tools to process and analyze big data. With the development and automation of modelling techniques, numerous advancements have been made in machine-learning techniques and algorithms. Data science allows for much more elaborate approaches and can be applied to numbers, text and images, thereby opening wide arrays of collection and analysis possibilities. Through its modernization initiative, Statistics Canada has been increasing the use of data science in many of its programs and is working toward a mainstreaming of these new techniques.
When the World Health Organization declared the COVID-19 outbreak to be a pandemic in March 2020, the need for timely information rocketed to levels previously unheard of. In a matter of days, due to the pressing demand for information on pandemic-related impacts, almost all traditional data collection approaches became insufficient. The pandemic highlighted the urgency of exploring and testing new methods and data sources. Thanks to its modernization initiative, Statistics Canada was well placed to respond. With an enhanced culture of innovation and the advent of modern methods, approaches that had been largely experimental (conceptual approaches or ones still at the testing and evaluation stages) became prime candidates to solve data needs. Data collection methods that had generally been viewed as options for the future were rapidly prototyped and implemented during the COVID-19 pandemic, for example:
- Web panels: This method uses a panel of individuals who will answer a series of questions online, either on a single occasion or several over time. Statistics Canada had already started to study the use of web panels by the time of the COVID-19 outbreak, so quickly rolled out this approach to collect timely social data. To introduce some elements of representativity to enable inference — and thereby linking traditional methods to modern approaches — the panel was constructed using respondents from the monthly Labour Force Survey who agreed to participate. Questions were on the knowledge and behaviour of Canadians regarding the pandemic.
- Crowdsourcing: Statistics Canada invited Canadians to voluntarily respond to online questionnaires posted on its website. This method was used to assess the impact of the pandemic on the Canadian population in areas such as mental health, perception of safety, trust in others, and disabilities.
- Web scraping: This technique gathers information directly from the internet, scraping data from webpages or other platforms. To respond to urgent needs for information on COVID-19 cases and on the basis of its expertise in economic statistics, Statistics Canada started to use web scraping to extract public information on case numbers from provincial and territorial websites, which enabled the production of frequent and timely national figures that could inform decision making by front-line agencies.
Although these data collection methods do have some limitations, given the context of the pandemic, they were deemed well within identified limits and conditions; their use was determined to be fit for purpose, provided that the limitations were well explained to users. In parallel, other methods were either implemented or their use significantly expanded; two important families of such methods are geospatial analysis tools and visualization methods. And, while it is important to innovate in the ways in which data are gathered, innovations also took place in aspects of the data life cycle. To grow the power of data, geospatial tools and techniques have increasingly been used to analyze and present information geographically in data hubs. Users can access data in the format they need to produce a wide variety of tailored statistics. Similarly, powerful tools are now being used to give information to users through tables and maps and to present information in a much more insightful and attractive way.
A Continuum of Options
During the past 75 years or so, NSOs have mainly focused on the use of probabilistic rather than non-probabilistic approaches. This choice is not a matter of preference; it has to do with their sound and proven theoretical framework, which enables statistical offices to not only produce statistics objectively but also to measure the precision of such statistics. In parallel, there exists a range of non-probabilistic approaches, which have been used in different settings such as clinical trials, observational studies and research involving opt-in groups or panels of various kinds. Many studies have been performed to assess and compare the two families of approaches, culminating in the 2013 report of the American Association for Public Opinion Research. The main difference between probabilistic and non-probabilistic approaches lies in their ability to lead to generalizations (inference) to the whole population of interest; probabilistic approaches enable their users to have control over the selection mechanism and thus have a smaller reliance on assumptions about this mechanism. There are situations in which the use of non-probabilistic methods is reasonable, and there are ways to combine them with probabilistic methods — as Jean-François Beaumont described recently in a comprehensive review — which can improve their potential for making inferences on the population being studied.
There is no such thing as a good or a bad method. Rather, a continuum of approaches exists, from a completely (or near-completely) controlled survey context, such as a census, at one end, to a completely ad hoc, arbitrary sampling of items, such as in web scraping, at the other. The quality of estimates produced by these methods depends not on the method itself but on how the method is implemented, what and how conclusions are drawn from it, and how these conclusions are communicated.
Figure 1: Continuum of Statistical Methods
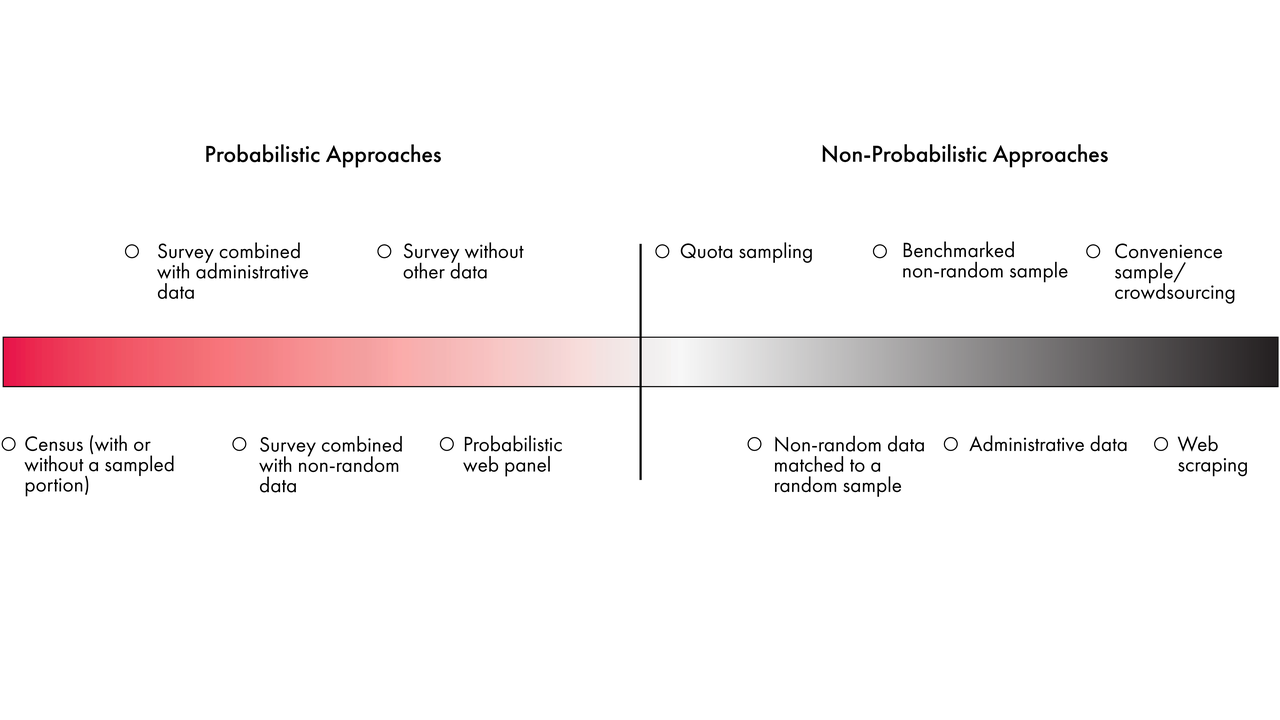
Figure 1 organizes several methods according to the strength of the assumptions required to support inference that can be made from them about the targeted population. The methods range from a complete enumeration of the population (a census), for which inference can obviously be made to the population, to methods such as crowdsourcing and web scraping, for which inference to the population either cannot be made or which require very strong assumptions about those who did not participate or about the information not obtained. More to the centre on the left-hand side are methods that use a selected sample and a probabilistic approach, while to the right-hand side are methods based on various forms of data sets or processes where the creation is not based on a probabilistic approach. Other factors that need to be considered include sample size, how the methods are implemented and, most importantly, their intended purpose. For example, considering inference and assumptions, small area estimation, a method that is concerned with producing estimates for domains with small or zero sample sizes and that makes use of both the sampling features and the model, would fall around the middle line.
Figure 2: Considerations in Using a Non-Probabilistic Method for Data Collection
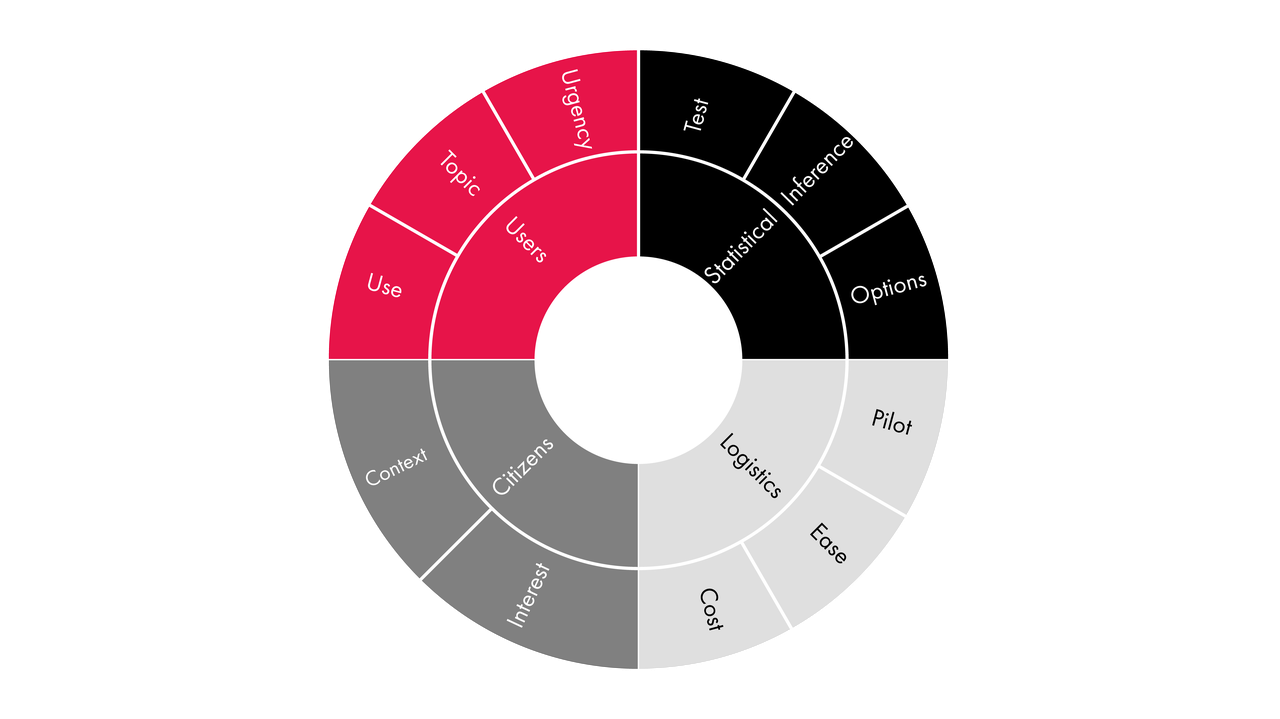
As one evaluates the context and need for methods that are fit for purpose, there are several elements that should be considered. First, one must consider the intended use of the statistics, the topic and urgency of the information needs.
Second, data collection always involves some form of citizen engagement, which means that context and public interest matter. For example, people are more willing to cooperate and provide information on the impact of COVID-19, say, than on other topics not as close to them. Further, there can also be privacy, ethical and social issues with data collection. This is why Statistics Canada developed its own Necessity and Proportionality Framework to provide a means to optimize collection while explicitly addressing such issues. New data acquisitions are assessed through the framework to account for potential data sensitivity, privacy requirements, options on alternative methods and the need to provide clear information in order to be fully transparent to Canadians.
Third, in terms of logistics, the cost of the collection method has a significant impact on decisions to develop a survey or to use other collection methods. Directly related to the cost is the ease or complexity of developing and implementing options. When the urgency is high and means are limited, simplicity becomes one of the most important factors to ensure the safe and sound gathering of data. To quickly obtain information from provinces on their COVID-19 case numbers, web scraping proved to be a very rapid and effective approach. Also, there may be a need to quickly pilot an approach to better understand the topic and context. Importantly, there are foundational statistical issues concerning coverage and bias and hence directly related to the need to make inference or not to the population of interest. Further, if other more precise statistical methods than the one contemplated exist, they should be seriously considered and weighed against the other conditions.
Finally, as part of the scientific approach, the objective could be to conduct a test to learn more about the methods before going forward with implementation. There could also be situations where there is no need to draw conclusions about the whole population, but rather to produce descriptive information about subgroups to provide a first insight. In this case, more methods could be considered along the continuum, while still ensuring all the desirable statistical features are taken into consideration.
Quality, Rigour and Transparency
No matter which method is used, assumptions will be required. Assumptions can be about coverage, about non-respondents, about measurement errors, about concepts used and understood. In all cases, efforts need to be made to attempt to validate as many assumptions as possible. Ideally, quality indicators can be produced to ensure that analysts and users are equipped with enough information to be able to draw the correct conclusions.
Probabilistic sampling approaches have the benefit of being supported by a sound and recognized theoretical framework. However, a rigorous development approach needs to be taken whether a probabilistic or a non-probabilistic method is adopted. The scientific approach provides this rigour and is conducive to innovation and research. As we have argued, in a data context in constant flux and with data needs emerging from all directions, NSOs have to be in the position to quickly respond to users’ needs. As such, ongoing and continuous and active research is required in all steps of the data life cycle, starting with all types of collection approaches. The importance of innovating and adapting methods to listen and respond to user needs has been long acknowledged, going back to Fellegi’s 1996 article presenting the desirable characteristics of effective statistical systems.
As methods are tested and adopted, it is very important to be proactive in keeping users and other Canadians well informed of methodological developments. For example, when new methods such as web panels are introduced, clear documentation is provided to users. Transparency is an important pillar of the statistical system. To this effect, the Statistics Act contains provisions for informing all Canadians — not just the minister of innovation, science and economic development — of new mandatory data collection initiatives, even if the authority to change methods lies in the chief statistician. Further, transparency is needed throughout the data life cycle to ensure data sensitivities are duly considered. Another aspect of transparency consists in informing users about quality and methods. Policies such as Statistics Canada’s Policy on Informing Users of Data Quality and Methodology have been put in place and are serving users well. Further, when non-probabilistic methods are used, it is important to inform users of limitations about the method itself, about how it was implemented, and about the limits within which conclusions can be made. As new methods are gradually introduced, one must deploy the means that will enable data literacy to follow suit.
Conclusion
There are no statistical collection tools that are absolutely right for all circumstances. Determining the users’ needs is the starting point, by clearly stating what the goals are and which public benefits are sought. We have seen that methods need to be fit for purpose and that there is a continuum of approaches. Given the appropriate conditions, one should select a method after carefully weighing its and others’ benefits and limitations. And, solutions are not limited to the use of one single approach. It is likely that new data demands will call for more than one method or a combination of approaches, wherein data will have to be integrated from more than one source. The methods all depend on the validity of the assumptions, which are stronger and harder to assess as one moves further into non-probabilistic territory.
As NSOs are producing official statistics, it is important that high-quality standards be used to measure social, economic and environmental aspects of society and that Canadians be provided with sound statistics. Paradoxically, although NSOs aspire to produce statistics in a stable and continuous fashion, they must constantly adapt their methods, because society is ever changing. As Walter Radermacher’s 2019 paper “Governing-by-the-numbers/Statistical Governance” makes clear, improvements and scientific developments will always be needed. That is why NSOs are heavily involved in research with their peers from around the world so as to be at the leading edge and ready with the capacity and agility to respond to fast-emerging demands. The COVID-19 pandemic has shown that innovations in data collection methods can happen in a sound scientific setting, even at a high pace. The use of non-probabilistic collection methods in NSOs is new, but with the right conditions, with rigour and a sound framework, they are increasingly becoming useful and could become essential tools in the official statistician’s tool kit.
Authors’ Note
The authors would like to thank Jean-François Beaumont, Hélène Bérard, François Brisebois, Sevgui Erman, Bob Fay, Susie Fortier and Michelle Marquis for their very helpful comments and, in particular, Martin Renaud, both for his comments and for the idea of a continuum of approaches.