Key Points
- Big-data-driven automated decision making expands and exacerbates discrimination. Policy addressing the role of big data in our lives will convey to the world how we, as a country, ensure the rights of the most vulnerable among us, respect the rights of Indigenous Nations and persist as a moral leader.
- To prevent big data discrimination, Canada’s national data strategy must address the challenge of biased data sets, algorithms and individuals; address the mismanagement of Indigenous data; and address the overarching challenge of consent failures.
- Canada must ensure the auditability and accountability of data sets, algorithms and individuals implicated in data-driven decision making. This should include efforts to protect Canadian data and decision-making systems from foreign interference, and efforts to ensure Canadian law governs Canadian data.
ig data presents Canada with a civil rights challenge for the twenty-first century.
The data-driven systems of the future, privileging automation and artificial intelligence, may normalize decision-making processes that “intensify discrimination, and compromise our deepest national values” (Eubanks 2018, 12).
The challenge is to check our innovation preoccupation, to slow down and look around, and say, we cannot allow big data discrimination to happen. Not here.
As the data-driven economy zooms forward — and along with it the desire for Canadian innovation and relevance — we must remember, as we rush toward could we, to always ask ourselves should we?
The policy determined in the coming years about the role of big data in our lives will speak volumes to how we, as a country, ensure the rights of the most vulnerable among us, respect the rights of Indigenous Nations and persist as a moral leader in the world. We cannot ignore these most important of responsibilities as we strive for innovation at home and recognition abroad.
The challenge is to check our innovation preoccupation, to slow down and look around, and say, we cannot allow big data discrimination to happen.
In a brief essay it is impossible to capture all that is being cautioned in the growing number of academic works with titles such as Automating Inequality (Eubanks 2018), Algorithms of Oppression (Noble 2018), Weapons of Math Destruction (O’Neil 2017), Broken Windows, Broken Code (Scannell 2016) and “Privacy at the Margins” (Marwick and boyd 2018a). The goal here is to acknowledge, as was noted in an aptly named Obama-era White House report entitled Big Data: Seizing Opportunities, Preserving Values: “big data analytics have the potential to eclipse longstanding civil rights protections in how personal information is used in housing, credit, employment, health, education, and the marketplace" (The White House 2014, 3).
To this list, we can add immigration, public safety, policing and the justice system as additional contexts where algorithmic processing of big data impacts civil rights and liberties.
The following section introduces overarching big data discrimination concerns. The second section outlines five big data discrimination challenges, along with policy recommendations to aid prevention. The final section provides a counter to the “new oil” rhetoric and a call to check the innovation preoccupation in light of the civil rights challenge.
Big Data Discrimination: Overarching Concerns
Automated, data-driven decision making requires personal data collection, management, analysis, retention, disclosure and use. At each point in the process, we are all susceptible to inaccuracies, illegalities and injustices. We may all be unfairly labelled as “targets or waste” (Turow 2012, 88), and suffer consequences at the bank, our job, the border, in court, at the supermarket and anywhere that data-driven decision making determines eligibility (Pasquale 2015). The quickly changing procedures for determining and implementing labels from myriad data points and aggregations must be scrutinized, as policy struggles to keep up with industry practice (Obar and Wildman 2015). (See Figure 1 for a list of problematic data broker labels identified by the US Senate.)
Figure 1: Data Broker Consumer Profile Categories
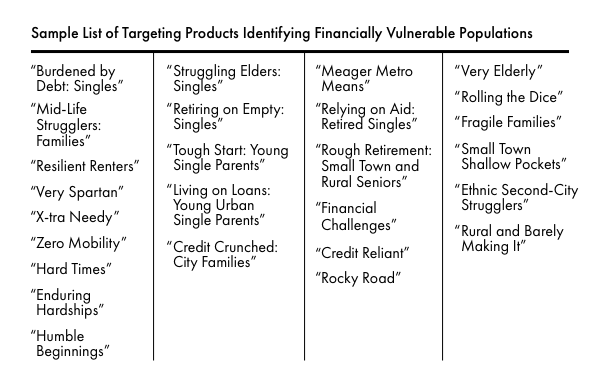
While this threatens us all, the research is clear: vulnerable communities are disproportionately susceptible to big data discrimination (Gangadharan, Eubanks and Barocas 2014; Newman 2014; Barocas and Selbst 2016; Madden et al. 2017). One revealing account comes from a study detailed in the recent book Automating Inequality, which identifies how these systems not only discriminate, but also reinforce marginality:
What I found was stunning. Across the country, poor and working-class people are targeted by new tools of digital poverty management and face life-threatening consequences as a result. Automated eligibility systems discourage them from claiming public resources that they need to survive and thrive. Complex integrated databases collect their most personal information, with few safeguards for privacy or data security, while offering almost nothing in return. Predictive models and algorithms tag them as risky investments and problematic parents. Vast complexes of social service, law enforcement, and neighborhood surveillance make their every move visible and offer up their behavior for government, commercial, and public scrutiny.
People of colour; lesbian, gay, bisexual, transgender and queer communities; Indigenous communities; the disabled; the elderly; immigrants; low-income communities; children; and many other traditionally marginalized groups are threatened by data discrimination at rates differing from the privileged (Marwick and boyd 2018b). Add to this the concern that vulnerability is not a static position, but one amplified by context. As Alice E. Marwick and danah boyd (ibid., 1160) argue, “When people are ill…the way they think about and value their health data changes radically compared with when they are healthy. Women who are facing the abuse of a stalker find themselves in a fundamentally different position from those without such a threat. All too often, technology simply mirrors and magnifies these problems, increasing the pain felt by the target…. Needless to say, those who are multiply marginalized face even more intense treatment."
In crafting a national data strategy, the government must acknowledge this quickly unfolding reality and ensure the protection of fundamental Canadian values.
Policy Recommendations: Preventing Big Data Discrimination
Addressing Discriminatory Data Sets
A common error in thinking about big data is that everything is new. The technology seems new, the possibility seems new and the data seems new. In reality, many of the historical data sets populated in health care, public safety, criminal justice and financial contexts (to name a few) are being integrated into new big data systems, and along with them, the built-in biases of years of problematic collection (see, for example, Pasquale 2015; Scannell 2016). At the same time, new data, whether flowing from millions of sensors and trackers or scraped from the data trails generated by lives lived online, may well perpetuate and amplify existing bias unless we actively guard against it.
Biased Policing, Biased Police Data
While so-called predictive policing based on big data analysis is relatively new in Canada, literature from the United States presents cautionary findings (Hunt, Saunders and Hollywood 2014; Angwin et al. 2016; Lum and Isaac 2016; Joh 2016; Scannell 2016). In particular, the concern that biased policing techniques (for example, broken windows policing, including stop and frisk1) contribute to biased police data. The use of historical data sets in new analyses, and the maintenance of biased policing techniques to generate new data, raise considerable concerns for civil rights in general, and automated criminal justice efforts in particular.
Canada is not immune to problematic policing practice2 and biased police data. For example, a Toronto Star analysis of 10 years’ worth of data regarding arrests and charges for marijuana possession, acquired from the Toronto Police Service, revealed black people with no criminal history were three times more likely to be arrested than white people with similar histories (Rankin, Contenta and Bailey 2017). Not coincidentally, this is similar to the rate at which black people are subject to police stops, or “carding” (ibid.).
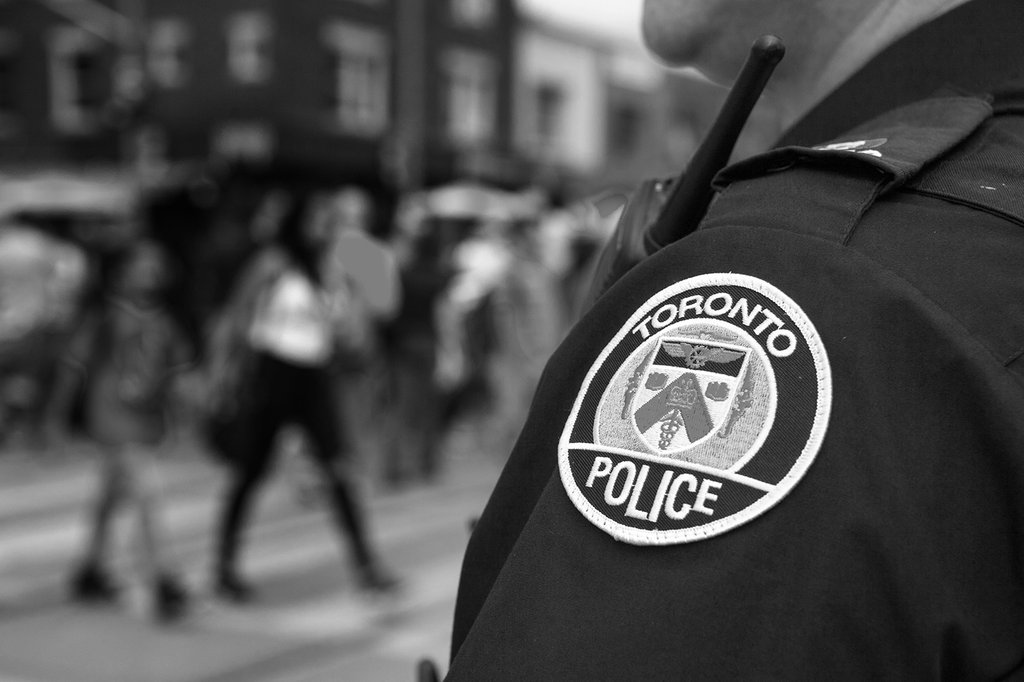
These findings were reinforced by statements from former Toronto Police Chief Bill Blair, who said, “I think there’s a recognition that the current enforcement disproportionately impacts poor neighbourhoods and racialized communities” (quoted in Proudfoot 2016). He later added that “the disparity and the disproportionality of the enforcement of these laws and the impact it has on minority communities, Aboriginal communities and those in our most vulnerable neighbourhoods” is “[o]ne of the great injustices in this country” (quoted in Solomon 2017).
To prevent big data discrimination, Canada’s national data strategy must acknowledge the challenge of biased data sets. Addressing this challenge might involve a combination of strategies for eliminating biases in historical and new data sets, being critical of data sets from entities not governed by Canadian law (see Andrew Clement’s contribution to this essay series) and developing policy that promotes lawful decision-making practices (i.e., data use) mandating accountability for entities creating and using data sets for decision making.
Addressing Discrimination by Design
Algorithms that analyze data and automate decision making are also a problem. Data scientist Cathy O’Neil now famously referred to biased algorithms wreaking havoc on “targets or waste” (Turow 2012, 88) as “weapons of math destruction” (O’Neil 2017). The scholarship is clear: writing unbiased algorithms is difficult and, often by design or error, programmers build in misinformation, racism, prejudice and bias, which “tend to punish the poor and the oppressed in our society, while making the rich richer” (ibid., 3). In addition, many of these algorithms are proprietary, so there are challenges in looking under the hood and toward ensuring public accountability (Pasquale 2015; Kroll et al. 2017).
To prevent big data discrimination, Canada’s national data strategy must acknowledge the challenge of biased algorithms. Addressing this challenge might involve a combination of strategies for auditing and eliminating biases in algorithms, being critical of algorithms from entities not governed by Canadian law and developing policy that promotes lawful decision-making practices (i.e., data use) mandating accountability for entities creating and using algorithms for decision making.
While a Canadian vision is necessary, international scholarship and policy initiatives may inform its development. American attempts to determine “algorithmic ethics” (see Sandvig et al. 2016) and to address algorithmic transparency and accountability (see Pasquale 2015; Kroll et al. 2017) may be of assistance. In particular, New York City’s legislative experiment, an “algorithmic accountability bill” (Kirchner 2017) might inform the development of oversight mechanisms.
Addressing People Who Want to Discriminate
Even if the data sets and the algorithms were without bias, some individuals might still want to discriminate. This old concern is also amplified at a time when digital tools aid and abet those circumventing anti-discrimination law in areas such as job recruitment (Acquisti and Fong 2015). This means that methods for protecting against big data discrimination must not just monitor the technology, but also the people interpreting the outputs.
To prevent big data discrimination, Canada’s national data strategy must acknowledge the challenge of biased individuals. Addressing this challenge might involve a combination of strategies for auditing and enforcing lawful data use, implementing what the Obama White House referred to as the “no surprises rule” (The White House 2014, 56) suggesting that data collected in one context should not be reused or manipulated for another, and being critical of decisions from entities not governed by Canadian law.
Addressing the Oppression of Indigenous Nations
The British Columbia First Nations Data Governance Initiative (2017) released a report in April 2017 identifying five concerns suggesting historical mismanagement of Indigenous data. These concerns are quoted below, updated with edits provided by Gwen Phillips, citizen of the Ktunaxa Nation and champion of the BC First Nations Data Governance Initiative:
It is equally important to recognize that nation states have traditionally handled and managed Indigenous data in the following ways: 1. Methods and approaches used to gather, analyze and share data on Indigenous communities has reinforced systemic oppression, barriers and unequal power relations; 2. Data on Indigenous communities has typically been collected and interpreted through a lens of inherent lack, with a focus on statistics that reflect disadvantage and negative stereotyping; 3. Data on Indigenous communities collected by nation state institutions has been of little use to Indigenous communities, further distancing Nations from the information; 4. Data on Indigenous communities collected by the nation state government has been assumed to be owned and therefore controlled by said government 5. With a lack of a meaningful Nation-to-Nation dialogue about data sovereignty.
The report also emphasizes the following recommendation: “The time for Canada to support the creation of Indigenous-led, Indigenous Data Sovereignty charter(s) is now. The Government of Canada’s dual stated commitment to the reconciliation process and becoming a global leader on open government presents a timely opportunity. This opportunity should be rooted in a Nation-to-Nation dialogue, with Indigenous Nations setting the terms of the ownership and stewardship of their data as it best reflects the aspirations and needs of their peoples and communities” (ibid.).
To prevent big data discrimination, Canada’s national data strategy must respect the rights of Indigenous Nations. How we address this issue should be viewed as central to our national ethics and moral leadership in the world. These concerns ought to be addressed in conjunction with, but also independently of, all other Canadian approaches to preventing big data discrimination.
Addressing Consent Failures
Consent is the “cornerstone” of Canadian privacy law (Office of the Privacy Commissioner [OPC] 2016). Consent is generally required for lawful data collection and use and, in theory, stands as a fundamental protection against misuse. There are two problems with the consent model as it relates to big data discrimination. First, current mechanisms for engaging individuals in privacy and reputation protections produce considerable consent failures. This is captured well by the “the biggest lie on the internet” anecdote — “I agree to the terms and conditions” (Obar and Oeldorf-Hirsch 2016). Scholarship suggests that people do not read, understand or even engage with consent materials. The growing list of reasons include: the length, number and complexity of policies (McDonald and Cranor 2008; Reidenberg et al. 2015), user resignation (Turow, Hennessy and Draper 2015), the tangential nature of privacy deliberation to user demands (Obar and Oeldorf-Hirsch 2016), and even the political economic motivations of service providers, manifested often via the clickwrap3 (Obar and Oeldorf-Hirsch 2017; 2018). Second, entities may not know how they want to use data at the time of collection, leading to vague consent provisions for retention, disclosure, research and aggregation (Lawson, McPhail and Lawton 2015; Clement and Obar 2016; Parsons 2017; Obar and Clement 2018). In this context, it is impossible for individuals to anticipate the ways their information might be used and re-used, never mind be aware of the potential for big data discrimination. In sum, when it comes to delivering privacy and reputation protections, while consent remains a strong place to start, it is also a terrible place to finish. On their own, consent mechanisms leave users incapable of challenging the complex threats expanded and exacerbated by big data (see Nissenbaum 2011; Solove 2012; Obar 2015; OPC 2016; 2017).
Consent is generally required for lawful data collection and use and, in theory, stands as a fundamental protection against misuse.
To prevent big data discrimination, Canada’s national data strategy must acknowledge the challenge of consent failures. Addressing this challenge might involve a combination of strategies for supporting new consent models and procedures at home and abroad, strengthening purpose specification requirements for data use, and ensuring lawful data use in all consequential data-driven decision-making processes, including eligibility determinations.
Canadian leadership should draw from extensive OPC consent consultations (OPC 2017), as well as from international efforts. In particular, in May 2018, the European Union will begin enforcing enhanced consent requirements through its General Data Protection Regulation.4 The requirements and outcomes should be evaluated as Canada develops its national data strategy.
Big Data Is Not the “New Oil”
So, no, big data is not the “new oil” for the Canadian economy. The beings whose bodies made the oil we burn died millions of years ago. You and I, and all persons in Canada, are not fuel — we are living human beings. Canada’s Charter of Rights and Freedoms grants us all “the right to the equal protection and equal benefit of the law without discrimination and, in particular, without discrimination based on race, national or ethnic origin, colour, religion, sex, age or mental or physical disability.”5
We must ensure that those who wield big data respect these rights. We must check our innovation preoccupation. Let us pursue policy with our eyes open, always with the goal of persisting as a moral leader in this world. We must protect the rights of the vulnerable. We must respect the rights of Indigenous Nations. We must prevent big data discrimination. That is the civil rights challenge of the twenty-first century.