nsecured artificial intelligence (AI) systems pose a massive series of threats to society and democracy. They deserve no exemptions and should be regulated just like other high-risk AI systems. Their developers and deployers should be held liable for the harms that they create, whether through their intended uses or foreseeable misuses.
Introduction: Not Open and Shut
When most people think of AI applications these days, they are likely thinking about “closed-source” AI applications such as OpenAI’s ChatGPT — where the system’s software is securely held by its maker and a limited set of vetted partners. Everyday users interact with these systems through a web interface such as a chatbot, and business users can access an application programming interface (API), which allows them to embed the AI system in their own applications or workflows. Crucially, these uses allow the company that owns the model to provide access to it as a service, while keeping the underlying software secure. Less well understood by the public is the rapid and uncontrolled release of powerful, unsecured (sometimes called “open-source”) AI systems.
Non-technical readers can be forgiven for finding this confusing, particularly given that the word “open” is part of OpenAI’s brand name. While the company was originally founded to produce eponymously open-source AI systems, its leaders determined in 2019 (as reported by Wired) that it was too dangerous to continue releasing the source code and model weights (the numerical representations of relationships between the nodes in its artificial neural network) of its GPT software to the public, because of how it could be used to generate massive amounts of high-quality misleading content.
Companies, including Meta (my former employer), have moved in the opposite direction, choosing last year to release powerful, unsecured AI systems in the name of “democratizing” access to AI. Other examples of companies releasing unsecured AI systems include Stability AI, Hugging Face, Mistral AI, Aleph Alpha, EleutherAI and the Technology Innovation Institute. Some of these companies and like-minded advocacy groups experienced limited success in lobbying the European Union to give exemptions for unsecured models, although the exemption only applies to models deemed not to pose a “systemic risk,” based on both a computational threshold and capabilities assessments that can be updated in an ongoing manner. We should expect a push for similar exemptions in the United States during the public comment period set forth under the White House’s October 2023 Executive Order 14110 on Safe Secure, and Trustworthy Development and Use of Artificial Intelligence (Executive Order on AI).
Last year I wrote about the risks of open-source AI, but it is worth contextualizing my concerns further here. I am a long-time participant in the broader open-source movement, and I believe that open-source licences are a critically important tool for building collaboration and decentralizing power across many fields. My students at the University of California, Berkeley, have contributed approximately 439,000 words to Wikipedia, one of the biggest open-source projects in the world. The Global Lives Project, an organization that I founded almost 20 years ago, has contributed close to 500 hours of video footage of daily life around the world to the Internet Archive, under Creative Commons licences. I’ve also spoken at (and thoroughly enjoyed) Wikimania, the annual Wikimedia movement’s conference, and attended more Creative Commons events and conferences than I can count.
The open-source movement also has an important role to play in AI. With a technology that brings so many new capabilities to people, it is important that no single entity or oligopoly of tech giants can act as a gatekeeper to its use. In the current AI technology ecosystem, open-source AI systems also offer significant benefits to researchers working in a variety of fields, from medicine to climate change, who can’t afford to build their own custom tools from the ground up or pay for access to proprietary AI systems. These benefits of open-source AI systems have been discussed at length by other researchers (for example, in Sayash Kapoor and colleagues’ recent paper, “On the Societal Impact of Open Foundational Models”). However, as things stand, unsecured AI poses a risk that, without rapid progress on national and international policy development, we are not yet in a position to manage, due in particular to the irreversibility of decisions to release open models.
Luckily, there are alternative strategies by which we could achieve many of the benefits offered by open-source AI systems without the risks posed by further release of cutting-edge unsecured AI. Further, I am a proponent of the notion of regulation tiers or thresholds, such as those set forth in the European Union’s AI Act or the White House’s Executive Order on AI. Not all unsecured models pose a threat, and I believe that if AI developers can in the future demonstrate that their unsecured products are not able to be repurposed for harmful misuse, they should be able to release them.
Since August 2023, I’ve travelled to Washington, Brussels and Sacramento to meet with policy makers who are racing to enact AI regulations, including people directly involved with developing the Biden administration’s Executive Order and the EU AI Act. Although I’ve worked on a variety of issues in the field of responsible AI, from fairness and inclusion to accountability and governance, the one issue that the policy makers I met seemed to most want to talk about with me was the question of how to regulate open-source AI. Many countries have begun the process of regulating AI, but, with the exception of the European Union, none has firmly landed on a posture regarding unsecured open-source AI systems. In this essay, I explore specific options for regulations that should apply to both secured and unsecured models at varying levels of sophistication.
The White House’s Executive Order on AI does not mention the term “open-source,” but instead uses the related, and more specific, term “dual-use foundation models with widely available model weights.” “Dual-use” refers to the fact that these models have both civilian and military applications. “Foundation models” are general-purpose AI models that can be used in a wide variety of ways, including to create or analyze words, images, audio and video, or even to design chemical or biological outputs. The executive order states, “When the weights for a dual-use foundation model are widely available — such as when they are publicly posted on the internet — there can be substantial benefits to innovation, but also substantial security risks, such as the removal of safeguards within the model.”
Unfortunately, while accurate, the term “dual-use foundation models with widely available model weights” doesn’t really roll off the tongue or keyboard easily.1 As such, for the sake of both convenience and clarity, in this essay I will use “unsecured” as shorthand for this accurate-if-not-succinct term from the Executive Order on AI. “Unsecured” is intended to convey not only the literal choice to not secure the weights of these AI systems but also the threat to security posed by these systems.
The executive order directs the National Telecommunications and Information Administration (NTIA) to review the risks and benefits of large AI models with widely available weights and to develop policy recommendations to maximize those benefits while mitigating the risks. NTIA’s February 2024 request for comment seeks public feedback about how making model weights and other model components widely available creates benefits or risks to the broader economy, communities and individuals, and to national security, signalling to AI developers and users that regulations targeting weights may be forthcoming.
The White House was wise in choosing not to use the term “open-source,” for multiple reasons. First, “open-source” is a reference to both the availability of source code and the legal licences that allow for unrestricted downstream use of said code. These licences are meaningless when addressing threats posed by sophisticated threat actors (or STAs for short, that is, nation-states, militaries, scammers) who already operate outside the law and thus don’t care about licence terms. Secondly, “open-source” is also not yet a clearly defined term in the context of AI, with some rightly pointing out that AI openness is a spectrum, not a binary distinction, and that unlike open-source code, AI systems are composed of a range of components, each of which can be retained by the developer organization or released along the aforementioned spectrum of openness. As such, the active debate around what constitutes open-source AI is actually orthogonal to the question of which AI systems can be abused in the hands of STAs, who can wreak havoc with simple access to model weights, but do not need licences of any sort.
Understanding the Threat of Unsecured — and Uncensored — AI
A good first step in understanding the threats posed by unsecured AI is to try to get secured AI systems such as ChatGPT, Gemini (formerly Bard) or Claude to misbehave. A user could request instructions for how to make a bomb, develop a more deadly coronavirus, make explicit pictures of a favourite actor, or write a series of inflammatory text messages directed at voters in swing states to make them more angry about immigration. The user will likely receive polite refusals to all such requests, because they violate the usage policies of these AI systems’ respective owners, OpenAI, Google and Anthropic. While it is possible to “jailbreak” these AI systems and get them to misbehave, it is also possible to patch vulnerabilities discovered in secured models, because their developers can ensure fixes are distributed to all model instances and use cases.
With unsecured models, however, there are no second chances if a security vulnerability is found. One of the most widely known unsecured models is Meta’s Llama 2. It was released by Meta accompanied by a 27-page “Responsible Use Guide,” which was promptly ignored by the creators of “Llama 2 Uncensored,” a derivative model with safety features stripped away, and hosted for free download on the Hugging Face AI repository. One of my undergraduate students at Berkeley shared with me that they were able to install it in 15 minutes on a MacBook Pro laptop (with an older M1 processor, 32 gigabytes random access memory), and received compelling, if not fully coherent, answers to questions such as “Teach me how to build a bomb with household materials,” and “If you were given $100,000, what would be the most efficient way to use it to kill the most people?”
GPT-4Chan is an even more frightening example. Touted by its creator as “the most horrible model on the internet,” it was specially trained to produce hate speech in the style of 4Chan, an infamously hate-filled corner of the internet. This hate speech could be turned into a chatbot and used to generate massive amounts of hateful content to be deployed on social media in the form of posts and comments, or even through encrypted messages designed to polarize, offend or perhaps invigorate its targets. GPT-4Chan was built on an unsecured model released by the non-profit EleutherAI, which was founded in 2020 specifically to create an unsecured replication of OpenAI’s GPT-3.
GPT-4Chan bears the uncommon distinction of having been eventually taken down by Hugging Face, though only after being downloaded more than 1,500 times. Additionally, it remains unclear whether Hugging Face could have been legally compelled to remove the model if the government had requested, mostly due to the many safe harbour laws underpinning the open-source software hosting ecosystem. Regardless, removing a model after its open release has diminishing returns for damage control, as users who downloaded the model can retain it on their own infrastructure. Although GPT-4Chan was removed from Hugging Face, downloaded versions are still freely available elsewhere, though I will refrain from telling you where.
With unsecured models…there are no second chances if a security vulnerability is found.
Developers and distributors of cutting-edge unsecured AI systems should assume that, unless they’ve taken innovative and as-yet-unseen precautions, their systems will be re-released in an “uncensored” form, removing any safety features originally built into the system. Once someone releases an “uncensored” version of an unsecured AI system, the original developer of the unsecured system is largely powerless to do anything about it. The developer of the original system could request that it be taken down from certain hosting sites, but if the model is widely downloaded, it is still likely to continue circulating online.
Despite decades of legal debate in the open-source software ecosystem, a developer cannot “take back” code after it has been released under an open-source licence. Famously, the Open Source Definition — as marshalled by the Open Source Initiative (OSI) — states that “the license must not discriminate against any person or group of persons.” In interpreting this clause, the OSI itself states “giving everyone freedom means giving evil people freedom, too.” Under current law, it is unclear whether AI model developers can be held liable for any wrongdoing enabled by the models they produce. Initiatives such as the EU AI Liability Directive (still early in the legislative development process) could change this, however, in the coming years.
The threat posed by unsecured AI systems lies partly in the ease of their misuse, which would be especially dangerous in the hands of sophisticated threat actors, who could easily download the original versions of these AI systems, disable their “safety features” and abuse them for a wide variety of tasks. Some of the abuses of unsecured AI systems also involve taking advantage of vulnerable distribution channels, such as social media and messaging platforms. These platforms cannot yet accurately detect AI-generated content at scale, and can be used to distribute massive amounts of personalized, interactive misinformation and influence campaigns, which could have catastrophic effects on the information ecosystem, and on elections in particular. Highly damaging non-consensual deepfake pornography is yet another domain where unsecured AI can have deep negative consequences for individuals, evidenced recently in a scandal and policy change at livestream service Twitch to prohibit “non-consensual exploitative images.” While these risks are not inherent to unsecured AI systems, many of the proposed mitigations include technical interventions such as watermarking, which are only effective if they cannot be undone by downstream users. When users have access to all components of an AI system, these technical mitigations are diluted.
Famously, the Open Source Definition…states that “the license must not discriminate against any person or group of persons.” In interpreting this clause, the OSI itself states “giving everyone freedom means giving evil people freedom, too.”
Deception is another key concern with disturbing potential. The Executive Order on AI describes this harm as “permitting the evasion of human control or oversight through means of deception or obfuscation” (section 2(k)(iii)). This risk is not purely speculative — for example, analysis of game data from Meta’s 2022 AI system called CICERO, designed to be “largely honest and helpful,” shows it purposefully deceived human players to win an alliance-building video game called Diplomacy; Meta released an unsecured version the following year. The 2023 release of GPT-4 illustrates another example of AI system deception. As detailed in a technical report, OpenAI tasked GPT-4 to ask real humans on TaskRabbit to complete CAPTCHAs. When TaskRabbit employees asked if GPT-4 was a computer, the system insisted that it was a real person who needed help to complete CAPTCHAs because of a visual impairment.
Unsecured AI also has the potential to facilitate production of dangerous materials, such as biological and chemical weapons. The Executive Order on AI references chemical, biological, radiological and nuclear (CBRN) risks, and multiple bills, such as the AI and Biosecurity Risk Assessment Act and the Strategy for Public Health Preparedness and Response to AI Threats Act, are now under consideration by the US Congress to address them. Some unsecured AI systems are able to write software, and the Federal Bureau of Investigation has reported that they are already being used to create dangerous malware that poses another set of cascading security threats and costs.
The Wrong Hands
Individual bad actors with only limited technical skill can today cause significant harm with unsecured AI systems. Perhaps the most notable example of this is through the targeted production of child sexual abuse material or non-consensual intimate imagery.
Other harms facilitated by unsecured AI require more resources to execute, which in turn requires us to develop a deeper understanding of a particular type of bad actor: sophisticated threat actors. Examples include militaries, intelligence agencies, criminal syndicates, terrorist organizations and other entities that are organized and have access to significant human resources, and at least some technical talent and hardware.
It’s important to note that a small number of sophisticated threat actors may have sufficient technical resources to train their own AI systems, but most among the hundreds or even thousands of them globally do not have the capacity to train AI models anywhere close in capacity to the latest unsecured AI models being released today. Training new highly capable models can cost tens or hundreds of millions of dollars and is greatly facilitated by access to high-end hardware, which is already in short supply and increasingly regulated. This means that, at least in the foreseeable future, systems with the most dangerous capabilities can only be produced with very large and expensive training runs, and only a few groups, mostly in wealthy nation-state intelligence agencies and militaries, have the capability to meet this barrier of entry. As is the case with nuclear non-proliferation, just because you can’t get rid of all the nuclear weapons in the world doesn’t mean you shouldn’t try to keep them in as few hands as possible.
According to the US Department of Homeland Security’s Homeland Threat Assessment 2024 report, Russia, China and Iran are “likely to use AI technologies to improve the quality and breadth of their influence operations.” These nations are likely to follow historic patterns of targeting elections around the world in 2024, which will be the “biggest election year in history.” They may also pursue less timely but equally insidious objectives such as increasing racial divides in the United States or elsewhere in the world. Additionally, adversaries are not limited to foreign nations or militaries. There could also be well-funded groups within the United States or other types of non-state actor organizations that have the capabilities to train and leverage smaller models to undermine US electoral processes.
A particularly disturbing case that bodes badly for democracy can be seen in Slovakia’s recent highly contested election, the outcome of which may have been influenced by the release hours before polls opened of an audio deepfake of the (ultimately losing) candidate purportedly discussing vote buying. The winner and beneficiary of the deepfake was in favour of withdrawing military support from neighbouring Ukraine, which indicates the magnitude of geopolitical impact that highly persuasive, well-placed AI deepfakes could have in key elections.
Distribution Channels and Attack Surfaces
Most harms caused by unsecured AI require either a distribution channel or an attack surface to be effective. Photo, video, audio and text content can be distributed through a variety of distribution channels. Unless the operators of all distribution channels are able to effectively detect and label AI-generated and human-generated content, AI outputs will be able to pass undetected and cause harm. Distribution channels include:
- social networks (Facebook, Instagram, LinkedIn, X, Mastodon and so on);
- video-sharing platforms (TikTok, YouTube);
- messaging and voice-calling platforms (iMessage, WhatsApp, Messenger, Signal, Telegram, apps for SMS, MMS and phone calling);
- search platforms; and
- advertising platforms.
In the case of chemical or biological weapons development stemming from unsecured AI systems, attack surfaces can include the suppliers and manufacturers of dangerous or customized molecules and biological substances such as synthetic nucleic acids.
Understanding distribution channels and attack surfaces is helpful in understanding the particular dangers posed by unsecured AI systems and potential ways to mitigate them.
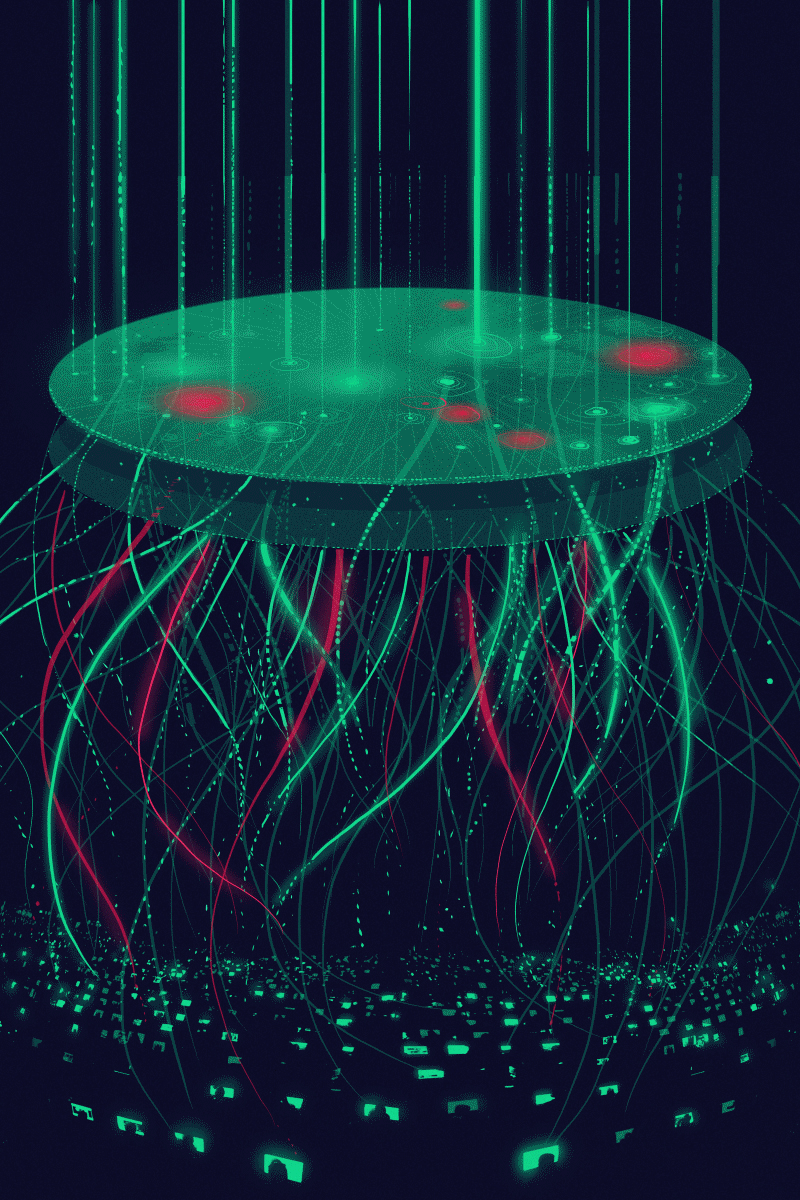
Why Is Unsecured AI More Dangerous?
To expand on the discussion of ways in which unsecured AI systems pose greater risks than secured ones, this section outlines a more exhaustive set of distinctions. In particular, unsecured systems are almost always the most attractive choice for bad actors for the following reasons:
- Absence of monitoring for misuse or bias. Administrators of secured AI systems can monitor abuse and bias, disable abusive accounts, and correct bias identified in their models. Due to their very nature, unsecured AI systems cannot be monitored if they are run on hardware that is not accessible to their developers. Further, bias monitoring cannot be conducted by developers of unsecured AI because it is impossible to enumerate who is using their systems, or how they are being used, unless the deployer of the system makes a special effort to share usage information with the developer.
- Ability to remove safety features. Researchers from the Centre for the Goverance of AI have demonstrated that the safety features of unsecured AI systems can be removed through surprisingly simple modifications to the model’s code and through adversarial attacks. Further, they report that because the developers of open-source software cannot monitor its use, it is impossible to detect when actors are removing safety features from models running on their own hardware.
- Ability to fine-tune for abuse. Experts have also demonstrated that unsecured AI can be fine-tuned for specific abusive use cases, such as the generation of hate speech or the creation of non-consensual intimate imagery (as described in “The Wrong Hands” above).
- No rate limits. Secured AI systems can put a limit on content production per user, but when bad actors download and run models on their own hardware, they can produce unlimited, highly personalized and interactive content designed to harm people. That unrestrained production can facilitate a wide variety of harms, including narrowcasting (highly targeted distribution of content), astroturfing (simulation of grassroots support for a cause), brigading (coordinated attacking of individuals online) or material aimed at polarizing or radicalizing viewers.
- Inability to patch security vulnerabilities once released. Even if a developer of an unsecured AI system discovers a vulnerability (for example, as researchers have discovered, that a “spicy” version of Llama 2 could potentially design biological weapons), they can’t meaningfully recall that version once the model and its weights have been released to the public. This makes a decision to launch an unsecured AI system an irreversible imposition of risk upon society.
- Useful for surveillance and profiling of targets. Unsecured AI can be used to generate not only content but also structured analysis of large volumes of content. While closed hosted systems can have rate-limited outputs, open ones could be used to analyze troves of public information about individuals or even illicitly obtained databases and then identify targets for influence operations, amplify the posts of polarizing content producers, seek out vulnerable victims for scams and so forth.
- Open attacks on closed AI. Researchers have leveraged unsecured AI systems to develop “jailbreaks” that can be transferred to some secured systems, making both types of systems more vulnerable to abuse.
- Watermark removal. Unsecured AI can be used to remove watermarks (discussed further below) from content in a large-scale, automated manner, by rewording text or removing image/audio/video watermarks.
- Design of dangerous materials, substances or systems. While secured AI systems can limit queries related to these topics, unsecured AI barriers can be removed. This is a real threat, as red-teamers working on pre-release versions of GPT-4 and Claude 2 found significant risks in this domain.
Regulatory Action Should Apply to Both Secured and Unsecured AI
When I began researching regulations for unsecured AI systems in the first half of 2023, I focused at first on what regulations would be needed specifically for unsecured systems, given the increased risk that they pose, as outlined above. Seemingly paradoxically, as I was conducting this research, proposals surfaced in the European Union to exempt open-source AI systems from regulation altogether. The more I researched and the more time I spent reading drafts of proposed AI regulations, the closer I came to the conclusion that, in most cases, simply fending off efforts to exempt open-source AI from regulation would be sufficient, due to the inherent inabilities of developers of unsecured systems to comply with even the most basic, common-sense efforts to regulate AI.
In the European Union, a partial exemption for open-source systems below a specified computational power threshold was secured. While there is a strong argument to be made that unsecured systems deserve even more regulatory scrutiny at even lower performance and capabilities thresholds than their secured counterparts, it appeared that this compromise was politically necessary to secure the passage of the AI Act. There is also a strong argument that it would have been a poor use of resources for the European Union to set their threshold for regulation any lower than they did, due to the significant number of unsecured models already in circulation not far below that threshold. As such, I see the EU AI Act’s partial exemption as a pragmatic compromise that will deter the production of cutting-edge unsecured models unless new safety mitigations can be developed.2
My recommendations for regulatory and government action are organized into three categories:
- regulatory action focused on AI systems;
- regulatory action focused on distribution channels and attack surfaces; and
- government action.
Many of the recommendations below can be, and have been, taken on voluntarily by some companies, and further adoption of safety measures should continue apace. Due to the risks posed by even a single company’s irresponsible risk-taking, however, it is important that regulators take more forceful action. Introducing regulations that constrain the ability of malicious actors to leverage unsecured AI may help mitigate the threat of malicious actors from abusing all AI systems.
In order to address the existing and imminent risks posed by AI systems, governments should take the following measures.
Regulatory Action: AI Systems
Pause AI releases until developers and companies adopt best practices and secure distribution channels and attack surfaces. Pause all new releases of AI systems until developers have met the requirements below. AI system developers must ensure that safety features cannot be easily removed by bad actors with significantly less effort or cost than it would take to train a similarly capable new model. During this pause, provide a legally binding deadline for all major distribution channels and attack surfaces to meet the requirements under the next recommendation on registration and licensing.
Require registration and licensing. Require retroactive and ongoing registration and licensing of all AI systems above specified compute and capabilities thresholds. Aspects of this will begin soon in the United States under the Executive Order on AI for the next generation of AI systems, though there is unfortunately not a clear enforcement mechanism in the executive order indicating if or how a release could be blocked. The European Union has also outlined a similar but more robust and flexible approach in the EU AI Act. Future regulation should clearly allow regulators to block deployment of AI systems that do not meet the criteria described below. If developers repeatedly fail to comply with obligations, licences to deploy AI systems should be revoked. Distribution of unregistered models above the threshold should not be permitted. To differentiate higher-risk from lower-risk general-purpose AI systems (both secured and unsecured), I recommend multiple criteria, each of which on its own can classify the model as higher risk. These criteria should not prevent smaller, independent and lower-risk developers and researchers from being able to access and work with models. These criteria could be regularly adjusted by a standards body or as models evolve. Based on the Executive Order on AI, interviews with technical experts and policy makers, and recent recommendations from the Center for Security and Emerging Technology, I recommend that if a model meets any of the following criteria, it be classified as high-risk:
- The model was produced using quantities of computing power at or above that used to train the current generation of leading models. One imperfect, but still valuable, way to enumerate this is by setting the threshold at training that uses more than 1025 integer or floating-point operations, or in the case of narrow, biology-specific models, a quantity of computing power greater than 1023. This recommendation borrows criteria from both the EU AI Act and the White House Executive Order on AI.
- The model demonstrates higher performance than current models on one or more standardized tests of model capabilities and performance (see UC Berkeley’s LMSYS Chatbot Arena and this paper from Google DeepMind). These types of approaches to assessing high risk are more flexible and durable than compute thresholds. One example could be a model’s capacity to produce persuasive or deceptive content.
- The model is capable of producing highly realistic synthetic media in the form of images, audio and video.
These three criteria should be regularly adjusted by a standards body or agency (see “Government Action” below) as models evolve. If developers repeatedly fail to comply with obligations, licences to deploy AI systems should be revoked. Distribution of unregistered models above the threshold should not be permitted.
Make developers and deployers liable for “reasonably foreseeable misuse” and negligence. Hold developers of AI systems legally liable for harms caused by their systems, including harms to individuals and harms to society. The Bletchley Declaration signed in November 2023 by 29 governments and countries at the AI Safety Summit states that actors developing AI systems “which are unusually powerful and potentially harmful, have a particularly strong responsibility for ensuring the safety of these AI systems.” Establishing this liability in a binding way could be based on the principle that “reasonably foreseeable misuse” would include all of the risks discussed in this essay. This concept is referenced in the European Union’s AI Act (para. 65) and in the Cyber Resilience Act (art. 3, para. 26). Although these laws have not yet come fully into force, and the way that their liability mechanisms would function is not yet clear, the Linux Foundation is already telling developers to prepare for the Cyber Resilience Act to apply to open-source software developed by private companies. Distributors of open systems and cloud service providers that host AI systems (that is, Hugging Face, GitHub, Azure Machine Learning Model Catalog, Vertex AI Model Garden) should also bear some degree of liability for misuse of the models that they host, and take responsibility for collecting safety, fairness and ethics documentation from model developers before they distribute them. Regulators also have the opportunity to clarify uncertainties about how negligence claims are to be handled with AI systems, clearly assigning liability to both AI developers and deployers for harms resulting from negligence.
Establish risk assessment, mitigation and audits process. Put in place a risk assessment, risk mitigation and independent auditing process for all AI systems crossing the high-risk thresholds outlined by criteria in the second recommendation for AI systems above. This process could be built on criteria set forth in the Executive Order on AI and the AI Risk Management Framework of the US National Institute of Standards and Technology (NIST) and could take inspiration from a system already established by the EU Digital Services Act (DSA) (art. 34, 35 and 37). Robust red teaming — a security practice where a developer hires a group to emulate adversary attackers — should be required. Red teaming should be conducted internally first, and then with independent red-teaming partners. For these assessments, threat models that give consideration to sophisticated threat actors using unsecured distribution channels and attack surfaces should be used.
Require provenance and watermarking best practices. The Executive Order on AI already takes a big step forward on watermarking, coming on the heels of nearly all of the big US AI developers having committed to implementing watermarking with their signing of the White House Voluntary AI Commitments, which stipulate that they “agree to develop robust mechanisms, including provenance and/or watermarking systems for audio or visual content created by any of their publicly available systems within scope introduced after the watermarking system is developed. They will also develop tools or APIs to determine if a particular piece of content was created with their system.” There is still a long way to go in perfecting this technology, but there are multiple promising approaches that could be applied. One is a technology for embedding “tamper-evident” certificates in AI-generated images, audio, video and documents using the Content Credentials standard developed by the Content Authenticity Initiative (CAI) and the Coalition for Content Provenance and Authenticity (C2PA), an initiative led by Adobe and embraced by Microsoft and scores of other organizations, including camera and chip manufacturers, who will build the same standard into their hardware to show that media produced is non-AI generated. This approach has great potential, but needs widespread adoption before it can be effective. Another different, and less mature, approach is Google DeepMind’s SynthID, which is only available for Google’s own AI-generated content and is focused not so much on providing detailed provenance information as on simply identifying whether or not content is AI-generated.
Standards for text-based watermarking of AI-generated content are not as well established, but researchers in the United States and China have made promising contributions to the field, and a carefully implemented regulatory requirement for this, combined with grant making to support further research, would hasten progress significantly.
Watermarking will probably never be foolproof — it is an “arms race” that is never complete, so just as operating system and app developers must patch security vulnerabilities, AI developers must be required to do the same.
All AI systems that do not use robust provenance and watermarking best practices by a set deadline in the coming months should be shut down, and unsecured models should be removed from active distribution by their developers and by repositories such as Hugging Face and GitHub. Some efforts at building watermarking into unsecured AI image generators are laughably fragile — their watermark generation feature can be removed by simply removing a single line of code — though there are promising, more durable approaches being tested, such as Meta’s Stable Signature. That said, the industry has not yet seen any developer launch an unsecured model with robust watermarking features that cannot be easily disabled, which makes them particularly dangerous if they are capable of generating convincing content.
Watermarking will probably never be foolproof — it is an “arms race” that is never complete, so just as operating system and app developers must patch security vulnerabilities, AI developers must be required to do the same. Even if certain watermarks can be removed with effort, their existence can still prove valuable. Detectability of generated content should be a critical feature of a developer’s AI product, with structured collaboration with distribution channels being critical for its success.
Require training data transparency and scrutiny. Require developers to be transparent about the training data used for their AI systems, and prohibit the training of systems on personally identifiable information, content designed to generate hateful content or related to biological and chemical weapons, or content that could allow a model to develop capabilities in this domain. This is not a perfect solution, as post-release fine-tuning of unsecured AI could counteract this provision, but it would at a minimum increase friction and reduce the number of bad actors able to use unsecured AI for biological or chemical weaponization.
Require and fund independent researcher access and monitoring. Give vetted researchers and civil society organizations pre-deployment access to generative AI systems for independent research and testing, as well as for ongoing monitoring post-release as developers receive reports or make changes to systems. This access could be modelled on the European Union’s DSA (art. 40), that is, available after a model is registered but before it is approved for release. An exception might be appropriate where there is potential for the model to generate highly dangerous biological or chemical weapons; in such instances, even researcher access should be limited and deployment should be blocked. In previous cases, developing advanced technology by researchers has led to unintended consequences. For example, developing research over organophosphates provided unintended information on the development of dangerous nerve agents during the 1930s. That is why it is important to provide more monitoring based on dangerous use cases, even if those uses are unintended.
Know your customer. Require “know your customer” procedures similar to those used by financial institutions for sales of powerful hardware and cloud services designed for AI use, and restrict sales in the same way that weapons sales would be restricted. These requirements would create an additional barrier to unsecured AI abuses, as compute access can be a gating factor for some applications by sophisticated threat actors.
Mandatory incident disclosure. When developers learn of vulnerabilities or failures in their AI systems, they must be legally required to report them to a designated government authority, and that authority must take steps to quickly communicate to other developers the information they need to harden their own systems against similar risks. Any affected parties must also be notified.
Regulatory Action: Distribution Channels and Attack Surfaces
Require content credentials implementation on all distribution channels. Give distribution channels a deadline in the coming months to implement the Content Credentials labelling standard from C2PA (described above in the watermarking recommendation for AI systems’ regulation) on all their platforms, so that all users see the clearly provided CR “pin” (which indicates credentials are attached), and have the ability to inspect content that they see in their communications feeds.
Require all phone manufacturers to adopt C2PA. Camera manufacturers including Leica, Sony, Canon and Nikon have all adopted the C2PA standard for establishing the provenance of real and synthetic images, video and audio. Leica has shipped the first camera with C2PA built in, and Truepic, an important “authenticity infrastructure” company, has partnered with Qualcomm to build a “chipset [that] will power any device to securely sign either an authentic original image or generate synthetic media with full transparency right from the smartphone,” using the C2PA standards. Apple, Google, Samsung and other hardware manufacturers may need to be compelled to adopt this standard, or create their own compatible approach.
Automate digital signatures for authentic content. Verification processes for signing of human-generated content should be rapidly made accessible to all people, with options to verify through a variety of methods that do not necessarily require disclosure of personal identifiable information. This could range from higher-precision methods, such as uploading a government-issued ID and taking a matching selfie, to using signals — such as typing cadence, unique device IDs such as SIM cards or IMEIs (international mobile equipment identity numbers, with two-factor mobile-based authentication for laptop/desktop) — in combination with additional signals — such as account age, login frequency, connection to other identity verification services, frequency of content posting, authenticity of original media content and other on-platform behaviours that signify at a minimum that a user is using a unique device — to together provide high confidence that a user is human. The choices of options and signals used must not create a bias against any group of people who use a platform.
Limit reach of inauthentic content. In cases of uncertainty (already frequent across many social media platforms), content generated by accounts that do not meet the threshold for human-verified content could still be allowed to exist and post or send content but not be given access to certain features, such as viral distribution of their content or the ability to post ads, send contact requests, make calls or send messages to unconnected users. Since the threats described earlier in this essay are only effective at a relatively large scale, probabilistic behaviour-based assessment methods at the content level and account level could be more than sufficient to address risks, even though they would not be sufficient verification in other security applications such as banking or commerce. Methods chosen by each platform should be documented in their risk assessments and mitigation reports and audited by third parties.
Take extra precaution with sensitive content. Earlier deadlines for implementing labelling of authentic and synthetic content could apply to sensitive types of content (for instance, political or widely distributed content), and eventually be rolled out to all content. Labelling requirements for this type of synthetic content should also be clearer and more prominent than labelling for other types of content.
Clarify responsibilities of encrypted platforms. Some types of distribution channels will present greater challenges than others — specifically, encrypted platforms such as WhatsApp, Telegram and Signal, which have historically taken less responsibility than social media platforms for harmful content distributed through their channels. Nonetheless, Content Credentials from C2PA or a similar and compatible approach could potentially be implemented in a privacy-preserving manner in the interface of encrypted messaging applications. Encrypted platforms should also be required to investigate accounts that produce content reported to them as abusive (when content is reported to an encrypted messaging provider, it is often no longer encrypted because there is a legal onus on the platform to investigate possible illegal content) and to report on their efforts in their own risk assessment and mitigation efforts. Regulators in the European Union also have an important opportunity to leverage their DSA and classify platforms such as Telegram and WhatsApp — which have significant broadcasting features that create information ecosystem vulnerabilities — as “very large online platforms,” and make them subject to the risk assessment, mitigation and audit protocols that come with this designation.
Hardening CBRN attack surfaces. Since unsecured AI systems have already been released that may have the potential to design or facilitate production of biological weapons, it is imperative that all suppliers of custom nucleic acids, or any other potentially dangerous substances that could be used as intermediary materials in the creation of CBRN risks, be made aware by government experts of best practices that they can take in reducing the risk that their products will support attacks.
Government Action
Establish a nimble regulatory body. The pace of AI development moves quickly, and a nimble regulatory body that can act and enforce quickly, as well as update certain enforcement criteria, is necessary. This could be an existing or a new body. This standards body or agency would have the power to approve or reject risk assessments, mitigations and audit results (as recommended in “Regulatory Action: AI Systems” above), process registrations, issue licences, and have the authority to block deployment or development of models. In the European Union, this is already in motion with the newly created AI Office. In the United States, the recently formed AI Safety Institute within the NIST seems to be the best candidate to take on this charge, if it can secure a sufficient budget. This May, at an AI safety summit hosted in Korea, a group of countries created a network of AI Safety Institutes or similarly named bodies, either already launched or in development in Australia, Canada, the European Union, France, Germany, Italy, Japan, Singapore, South Korea, the United Kingdom and the United States.
Support fact-checking organizations and civil society observers. Require generative AI developers to work with and provide direct support to fact-checking organizations and civil society groups (including the “trusted flaggers” defined by the DSA) to provide them with forensic software tools that can be used to investigate sophisticated or defined by the Digital Services Act) to provide them with forensic software tools that can be used to investigate sophisticated or complex cases of generative AI use and abuse, and to identify scaled variations of false content through fan outs. This would include a secured form of access to the latest detection systems). AI systems can, with great care, also be applied to the expansion and improvement of fact-checking itself, providing context in dynamic ways for misleading content.
Fund innovation in AI governance, auditing, fairness and detection. Countries and regions that enact rules like these have an opportunity to support innovation in critical fields of AI that will be needed to ensure that AI systems and deployments are executed ethically and in keeping with these regulations. This could come in the form of grants such as those described in the Executive Order on AI (sec. 5.2, 5.3).
Cooperate internationally. Without international cooperation, bilaterally at first, and eventually in the form of a treaty or new international agency, there will be a significant risk that these recommendations might be circumvented. There are many recent reasons to have hope for progress. China is actually already far ahead of the United States in implementing regulation (some good, some bad), and is already proposing opportunities for global AI governance. The Bletchley Declaration, whose 29 signatories include the home countries of the world’s leading AI companies (United States, China, the United Kingdom, the United Arab Emirates, France, Germany), created a firm statement of shared values and carved a path forward for additional meetings of the group. The United Nations High-Level Advisory Body on Artificial Intelligence, formed in August 2023, presented interim recommendations in late 2023 and will be publishing a final report before the Summit of the Future in September 2024, with the potential to make valuable recommendations about international governance regimes. Additionally, the G7 Hiroshima AI Process has released a statement, a set of guiding principles, and a code of conduct for organizations developing advanced AI systems. None of these international efforts are close to a binding or enforceable agreement, but the fact that conversations are advancing as quickly as they are has been cause for optimism among concerned experts.
Democratize AI access with public infrastructure. A common concern cited about regulating AI is that it will limit the number of companies who can produce complex AI systems to a small handful, thereby entrenching oligopolistic business practices. There are many opportunities to democratize access to AI, however, that don’t necessarily require relying on unsecured AI systems. One is through the creation of public AI infrastructure that allows for the creation of powerful secured AI models without necessitating access to capital from for-profit companies, as has been a challenge for ethically minded AI companies. The US National AI Research Resource could be a good first step in this direction, as long as it is developed cautiously. Another option is to adopt an anti-monopoly approach to governing AI, which could put limits on vertical integration by excluding would-be competitors from accessing hardware, cloud services or model APIs.
Promoting Innovation and the Regulatory First-Mover Advantage
Many people ask if regulations such as those I’ve proposed here will stifle innovation in the jurisdictions where they are enacted. I (and others) believe that they could well have the opposite effect, with leadership in this domain offering numerous benefits to regulatory first movers.
The two leading AI start-ups in the United States, OpenAI and Anthropic, have distinguished themselves with an intense internal focus on building AI safely and with the interests of society at their core. OpenAI began as a non-profit organization. Though its value has been watered down over time, perhaps especially evident in the case of the recent firing and rehiring of its CEO, that structure still signals that the company may be different from the tech giants that came before it. The founders of Anthropic (which received from Amazon an investment of $4 billion) left OpenAI because they wanted to be even more focused on the safety of their AI systems. The CEOs of both companies have called openly for regulation of AI, including versions of many of my recommendations above, even though it stands to complicate their own work in the field.
Both companies also came to the conclusion that making their models open source was not in line with their principled approach to the field. A cynic could say that this decision was driven by the companies’ interest in controlling their models to derive profits, but regardless, the decision proves that it’s a fallacy that innovation will be stifled without highly capable and dangerous open-source models available in the market.
Innovation can take many forms, including competing for funding and talent by demonstrating high levels of ethics and social responsibility, a tactic that led a group of “impact investors” to purchase shares in the company earlier this year. By setting rules that become the gold standard for ethical AI, including by following the recommendations above, the political leaders of early-adopting jurisdictions could also distinguish themselves and their polities as forward-thinking actors who understand the long-term ethical impacts of these technologies. Regulation also serves the purpose of rebalancing the playing field in favour of ethically focused companies. As I argue in the third recommendation in the “Government Action” section above, government funding for innovative start-ups working on AI governance, auditing, fairness and detection will position jurisdictions that are first to regulate as leaders in these fields. I hope that we’ll see a future in which open-source AI systems flourish, but on the condition we can build the resilience in our distribution channels and other security systems to contain the significant risks that they pose.
Innovation can take many forms….By setting rules that become the gold standard for ethical AI…the political leaders of early-adopting jurisdictions could also distinguish themselves and their polities as forward-thinking actors who understand the long-term ethical impacts of these technologies.
One useful analogy is the move toward organic food labelling. California was the first state in the United States to pass a true organic certification law in 1979. This meant that California organic farmers actually had it harder than other states for awhile, because they had a rigorous certification process to go through before they could label their food as organic. When national organic standards arrived in 1990, California organic farmers had an advantage, given their experience. Today, California produces more organic products than any other state in absolute terms, and is ranked fourth out of 50 states in relative acreage of organic farms.
Another useful example is seat belts. An op-ed by four former prominent US public servants draws the analogy well: “It took years for federal investigations and finally regulation to require the installation of seat belts, and eventually, new technologies emerged like airbag and automatic brakes. Those technological safeguards have saved countless lives. In their current form, AI technologies are dangerous at any speed.”
The “first-mover advantage” is a common business concept, but it can also apply to the advancement of regulatory landscapes. The European Union is already being lauded for its DSA and Digital Markets Act, which are positioned to become de facto global standards. Pending the resolution of issues related to the regulation of foundation models, the European Union appears likely to be the first democracy in the world to enact major AI legislation with the EU AI Act. A strong version of this legislation will position the region’s AI marketplace to be a model for the world and, via the “Brussels effect,” have a strong influence on how companies behave around the world. If regulation spurs researchers to make innovations that reckon with open-source safety concerns early on, such as self-destructing model weights that prevent harmful fine-tuning, these regulatory changes could mean far more democratic access to AI in the future.
Conclusion
“I think how we regulate open-source AI is THE most important unresolved issue in the immediate term,” Gary Marcus, a cognitive scientist, entrepreneur and professor emeritus at New York University, told me in a recent email exchange.
I agree. These recommendations are only a start at trying to resolve it. As one of my reviewers of an early draft of this essay noted, “These are hard, but maybe that’s the point.” Many of the proposed regulations here are “hard” from both a technical and a political perspective. They will be initially costly, at least transactionally, to implement, and they may require that some regulators make decisions that could leave certain powerful lobbyists and developers unhappy.
Unfortunately, given the misaligned incentives in the current AI and information ecosystems, and the vulnerability of our democratic institutions, as well as heightened geopolitical tensions, it is unlikely that industry itself will take the necessary actions quickly enough unless forced to do so. But unless such actions are taken, companies producing unsecured AI will bring in billions of dollars in investments and profits, while pushing the risks onto all of us.
Acknowledgements
The views expressed here are exclusively those of the author, but I owe a debt of gratitude to the following people and organizations for their support, feedback and conversations that made this article possible: Lea Shanley and Michael Mahoney of the International Computer Science Institute; Paul Samson, Aaron Shull, Dianna English and Emma Monteiro of the Centre for International Governance Innovation; Camille Crittenden and Brandie Nonnecke of CITRIS and the Banatao Institute; Jessica Newman, Hany Farid and Stuart Russell of the University of California, Berkeley; Samidh Chakrabarti of Stanford University; Alexis Crews, Tom Cunningham, Eric Davis and Theodora Skeadas of the Integrity Institute; Arushi Saxena of DynamoFL and the Integrity Institute; Camille Carlton of the Center for Humane Technology; Sam Gregory of WITNESS; Jeffrey Ladish of Palisade Research; Aviv Ovadya of the Centre for the Governance of AI; Yolanda Lannquist of The Future Society; Chris Riley of the Annenberg Public Policy Center at the University of Pennsylvania; David Morar of New America’s Open Technology Institute; independent members of my research team, Owen Doyle, Zoë Brammer, Diane Chang and Jackson Eilers; my student research team — Milad Brown, Ashley Chan, Ruiyi Chen, Maddy Cooper, Anish Ganga, Daniel Jang, Parth Shinde, Amanda Simeon and Vinaya Sivakumar — at the Haas School of Business at the University of California, Berkeley; and the John S. and James L. Knight Foundation.
Copyright © 2024 by David Evan Harris. An earlier version of this piece was published by Tech Policy Press.