Key Points
- Canada is well positioned to dominate the world landscape in applying big data and machine learning to its biggest primary industries.
- Canada has significant advantages: the largest, best-instrumented and most modern primary industries; world-leading subject matter experts; and top university graduates.
- The historical data collected in Canada’s industries is not suitable for the new big data methods, but the expensive part of the infrastructure is already in place and can be used for the collection of the new type of data that is required — this can be done rapidly and cheaply.
- Once this data is collected, Canada will have all of the ingredients for a renaissance in its biggest industries and the potential for large exports of the expertise that will be developed; however, if we do not seize the opportunity, Canada risks being left behind.
avid Thompson, an explorer and cartographer who mapped most of western and parts of eastern Canada as well as the northern United Sates in the late 1700s and early 1800s, has been called the greatest land geographer who ever lived. Thompson travelled approximately 90,000 km by foot and canoe and used the data he collected to create what he called the “great map”— the most complete record of the territory of more than 3.9 million km2.1 His map unlocked the commercial potential of North America.
Big data is as important to Canada in the twenty-first century as Thompson’s topographical data was in the nineteenth century. It has the potential to redefine Canada’s contemporary commercial and environmental landscape. Big data is a term that describes the large volume of data that now inundates the world. According to IBM, in 2013, 2.5 exabytes — that is, 2.5 billion gigabytes — of data was generated daily (Wall 2014). Data continues to accumulate so quickly that approximately 90 percent of it has been collected in just the past two years (Marr 2015). This data comes from everywhere: sensors used to gather shopper information or industrial machinery performance; posts to social media sites; digital pictures and videos; purchase transactions; and cellphone global positioning system signals, to name a few.
It is not the amount of data that is important, but what is done with it. It is the "great map" data scientists and machine learning specialists can make from the data.
The consulting firm Bain & Company demonstrated the significance of data by examining more than 400 large companies and found that those with the most advanced analytics capabilities were outperforming competitors by wide margins. They were:
- twice as likely to be in the top quartile of financial performance within their industries;
- five times as likely to make decisions faster than market peers;
- three times as likely to execute decisions as intended; and
- twice as likely to use data very frequently when making decisions (Pearson and Wegener 2013).
It is obvious that the combination of big data with modern machine learning will unlock new commercial opportunities and significantly reduce the environmental impacts of Canada’s biggest industries through continued optimization and by identifying and solving new problems and challenges. We can do more with less and we can do it better using the new techniques to find overlooked opportunities. Using the right type of data, machines can find opportunities for improvements that are not obvious to humans.
Google, Facebook and Amazon dominate the consumer big data space and they have proven that data-driven improvements can have an impact on every aspect of our lives. Big data and machine learning have generated significant improvements in productivity and new ways of doing things across a range of consumer applications. So far, largely due to a lack of quality data, these techniques have not been broadly applied to primary industry. It is the one area of big data where Canadians are not at a disadvantage due to our smaller population.
For the last century, Canada has led the world in the primary industries: mining, energy, forestry and agriculture. For the most part, the focus has been on digging, cutting and planting followed by selling after primary processing.
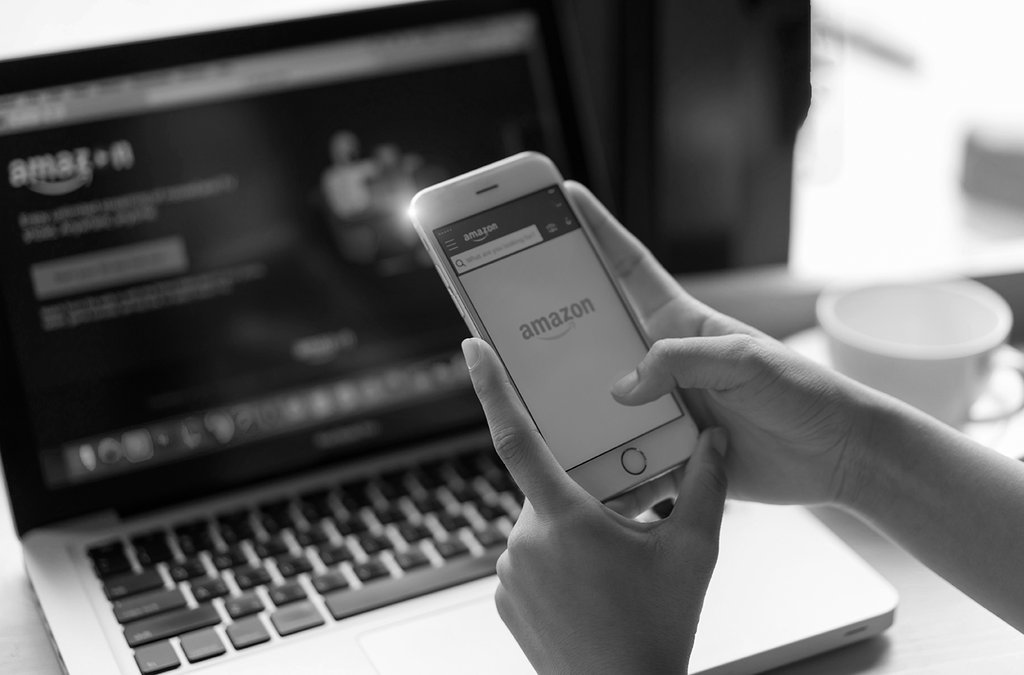
Not Just Big Data, but a Big Opportunity
Applying big data to our primary industries means lower costs and reduced environmental impacts: less waste, emissions and land disturbance while creating the valuable new-economy jobs that will define Canada’s success in the next 100 years.
Although Canadian universities produce a disproportionate share of the world’s big data experts, Canada ranks poorly in big data opportunities. The country’s small population means that consumer-related potential is small. Many of the best and brightest big data experts leave for the United States because there are not opportunities for them in Canada.
Population is not a disadvantage for Canada when looking for opportunity in primary industries. Canada is more reliant on, and has more opportunity in, primary industry than other Group of Seven countries. Primary industries are important contributors to Canadian employment, capital investment, exports and GDP. The scale and modernity of Canada’s industries is a competitive advantage. We also have world-leading subject matter expertise, an essential ingredient in finding opportunity when working with the machine learning methods that rely on big data.
Ironically, Canada does not have the right type or quantity of data to enable these new big data opportunities. The opportunity — the “big idea” — is to enable transformative change by collecting and cataloguing the right data for rapid application of machine learning and artificial intelligence (AI) to our biggest primary industries.
It is not the amount of data that is important, but what is done with it.
This should start with a pilot program to establish the infrastructure and begin populating what will eventually become a large open-source data library for primary industry. Once we learn by trying, we can rapidly advance to allow Canada to fill the rest of the library and become the world leader in the emerging space of primary industry big data.
The reasons for the paucity of data date back to the 1960s, when primary industries around the world started using computers to collect data and for measurement and process control. The sensors2 they used were connected to computers using a system called SCADA (Supervisory Control and Data Acquisition). The SCADA protocol, which is now ubiquitous, enabled communication between a computer and a remote sensor or control device. Simple examples would be to request a temperature or pressure reading, or to remotely operate a valve. The amount, type and contextualization of data that is now routine for big data were unknown at the time SCADA was conceived. Although many improvements have been made since the 1960s, SCADA and SCADA-like systems are simply not adequate for this big data job, for a number of reasons:
- SCADA is essentially a serial connection from the computer to the sensor, the computer phones the sensor and records a reading, and progresses to the next sensor (between calls the data is not available, if you call at the wrong time you miss things of interest);
- the sensors do not have intelligence — they cannot select what to record or how much to save and does not have any ability to encrypt or compress the data;
- the communication methods are antiquated and expensive; and
- the systems were not designed for really large quantities of data.
Using the SCADA systems for big data applications is like trying to develop a self-driving car with the data from the back-up beeper.
With the arrival of the Internet of Things, there are proven low-cost options to help solve the SCADA problem.
Imagine: Parallel Communication, Intelligent Data Collection and Open-source Organization
Canada controls the important landscape required to build the “Facebook of sensors” for primary industry:
- Imagine if the SCADA-connected sensors in the mining, energy, forestry and agriculture sectors could be “woken up” by installing a low-cost communication and smart data collection system3 in parallel, at the sensor, with the existing SCADA system.
- Imagine if that system could collect multivariate, real-time data that could be transmitted and stored in the format required for big data while continuing to allow the SCADA system to operate as intended.
- Imagine if data could be collected from the millions of sensors that are in Canada’s primary industries. This data would enable application of the new techniques to be applied broadly and the types of improvements that have been demonstrated in the consumer space to occur in Canada’s primary industries. Improvements in these industries do not just reduce costs on increased throughput, large-scale environmental improvements occur concurrently because the impact for each unit of output is being reduced.
- Imagine if this data was collected from the start with the end in mind, following a plan conceived by big data experts and subject matter experts working together. Time would not be wasted in trying to clean up the wrong type of data — what is needed would be collected from the start.
- Imagine if this data was open source and broadly available in an ecosystem created so that Canada’s best and brightest young minds could collaborate with experienced subject matter experts from industry to find and exploit the best opportunities.
- Imagine if Canada’s existing large industries provided the commercial opportunities to keep our best and brightest at home.
Parts of this future are already happening. In March 2017, the UK National Grid announced a partnership with a Google-owned AI company called DeepMind. The goal is to collect real-time operational data about the supply and demand choices of energy customers. This data would then be used to develop algorithms to increase efficiency through better integration of generation from intermittent sources such as solar and wind. Grid officials estimate that they could reduce the need for new generation by up to 10 percent (Murgia and Thomas 2017).
One Other Important Reason Why Canada Can Lead
Compared to the rest of the world, Canada’s primary industries are modern and well instrumented. It has been estimated that there are more than five million sensors in Canada’s industries, at an installed cost of more than $10,000 each. That’s $50 billion of sensors. Historically, although lots of data has been collected from these sensors, this historical data is just not suitable for the new big data and machine-learning methods. That is easy and inexpensive to change.
The opportunity — the “big idea” — is to enable transformative change by collecting and cataloguing the right data for rapid application of machine learning and AI to our biggest primary industries.
The sensors — the expensive part — are already in place. Technology is available to put robust, secure communications and small amounts of computing power and storage right at the sensor on “the edge.” This allows the extra, currently unused, measuring capacity in the sensor to be utilized to collect what is needed. It is analogous to the unoccupied residential rooms rented through Airbnb: the sensors are sitting there unoccupied and can be used at very low cost.
The “edge” computer can collect the large volume and type of data from the existing sensor, and then send it directly (and securely) to the cloud. The SCADA system can continue to operate without interruption.
The new data will be collected and organized from the start to be immediately useful for big data methods and because it is already being done on a limited basis in parts of the Canadian energy industry, it will be at low technical risk.
Benefits can be both big and fast. Achieving major environmental benefits usually requires new processes and substantial investment with high adoption risks and lengthy time frames. Big data improvements do not require new process development or facilities — in existing industries, improvements are big because the industries are big, and they are fast because the facilities are already there. They do not require much capital, as they result from finding additional capacity through new ways of operating. The new big data entrepreneurs are looking for commercial opportunity and the data to exploit it, and will stay home, in Canada, if they get what they need here.
Security
Any discussion on big data seems to default to security right after the discussion of the potential benefits. There is a continuum of security-related concerns: at the high end is individual medical data and at the low end is the reading on a temperature gauge. Industrial security concerns are important, but they can be solved more easily than situations involving the collection of data on individuals. Most industrial security concerns can be mitigated by keeping initial collection efforts at the individual item of equipment level, by the anonymization of the data collected and by a user-controlled period of latency before the data becomes publicly available.
Different levels of security are and will be required for different types of data. Conducting pilot programs for industrial applications, where the sensitivities are lower, is the place to start. What is learned regarding security and confidentiality will provide guidance for other areas, which are likely to require higher standards and protocols.
The Canadian Way: Access and Innovation
So far, efforts in big data in the primary industry space have been by dominant industrial players collecting proprietary data to improve their competitive position. For example, John Deere collects self-driving tractor information, and GE collects gas turbine maintenance information. Their commercial strategy sees value in keeping this data proprietary.
What is missing in the National Grid DeepMind project and other examples from the imagined future is a vision for public accessibility of big data (with suitable authorization access to ensure appropriate protections for security and privacy) to accelerate and unleash broader, continuous, cross-sectoral innovation. To produce broad benefits for Canadians, this data must be intelligently organized and stored, and made available on an open-source basis, like the libraries of old.
Canada is well positioned to take a leadership role in the creation of such a library, by bringing together the know-how it has fostered in its primary industries and its emerging leadership in machine learning and data science.
Canada has an historical example that is unique in the world regarding the success of an open-source library for primary industry.
The transfer of mineral rights from the federal government to Alberta after the discovery of the Turner Valley oil field south of Calgary in 1914 led to the establishment of what has now become the Alberta Energy Regulator (previously called the Energy Resources Conservation Board). One of the board’s first actions was to require public reporting of key attributes of production, geology and reservoir performance, which formed the basis for a comprehensive historical library on Alberta’s resources. Everything related to well performance and reservoir is recorded and becomes public after a one-year period following drilling.
An unintended, but beneficial, consequence of this early idea for public reporting and archived information was to lower barriers to entry for oil industry entrepreneurs. Free public access to what had traditionally been proprietary data spawned large-scale resource development in a competitive environment in Alberta that continues to this day.
The public model developed in Alberta has now been recognized as a key enabler of the rapid and continuing entrepreneurial development in Alberta as well as a best-in-class model for petroleum resource regulation in other areas of the world.
The great libraries of the past point the way to the future: the greatest benefits and opportunities for Canadians will be achieved if the data is open access and available to all.
What Is the Rush?
It is important to remember that this is not a static situation. Some of the main multinational equipment suppliers are already starting to collect proprietary data that will lead their development of intellectual property and control of parts of the space. Canada currently has important advantages but cannot be lethargic. We must lead aggressively or our competitive advantage will be lost.
The greatest benefits and opportunities for Canadians will be achieved if the data is open access and available to all.
The approach of big data incumbents in the consumer world seems to be to collect everything they can and worry about the policy when they encounter pushback. They develop policy after the data is collected.
If less-sensitive data is targeted as a starting point, for example, readings on a pressure gauge, the policy can be developed concurrently with pilot programs in less-sensitive areas. The pilot approach will allow the identification of issues that may help to inform policy in more sensitive areas.
Pilots can begin while policy evolves so that Canada’s advantage is not lost.
The Twenty-First-Century Great Map
Imagine big data in an open-source library for primary industry, conceived from inception, to stimulate opportunity for Canada’s new generation of big data entrepreneurs.
By collecting the raw data and making it open source, new big data businesses will be built and sustained in Canada, enticed by the three essential ingredients for success: the right type of data; the subject matter experts who can help identify pressing problems; and a large domestic market.
If the organizational structure is developed to link young Canadian big data professionals with Canada’s deep industry expertise and support them to found new enterprises, primary industry in Canada and around the world can be revolutionized.
These young professionals can draw Canada’s next great map.
Concurrently, a big data entrepreneurial ecosystem system must be developed that will encourage Canada’s best and brightest to pursue these data-driven opportunities at home, rather than leaving for opportunities south of the border. This ecosystem should provide managerial support for new data-driven businesses, together with small amounts of capital for new ideas that have merit.